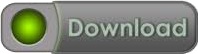
Furthermore, little work is available on drift detection for high-dimensional supervised data streams. Although they can detect some drifts within the input space, changes affecting only the labelling mechanism cannot be identied. Another category of drift detectors are data distribution-based detectors. This may not be sufcient in many application areas where the reason behind a drift is also important. Most drift detection methods for supervised data streams are performance-based, that is, they detect changes only after the classication accuracy deteriorates. In a streaming environment, the characteristics of the data themselves and their relationship with the labels are likely to experience changes as time goes on. Experiments show the effectiveness of the proposed approach both on synthetic dataset and measurements from real applications. This hierarchical solution significantly reduces the number of false positives at the expenses of a negligible increase of false negatives and detection delays. Following this approach, we suggest a novel two-level hierarchical change-detection test designed to detect possible occurrences of changes by observing incoming measurements. Moreover, such tests can be made automatic through an on-line inspection of sequences of data, hence making them particularly interesting to address real applications. In this direction, the literature provides several tests aiming at assessing the stationarity of a data generating process of particular interest are nonparametric sequential change-point detection tests that do not require any a-priori information regarding both process and change. Design of applications working in nonstationary environments requires the ability to detect and anticipate possible behavioral changes affecting the system under investigation.
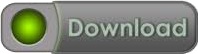